How to Get Started with AI for Your Business
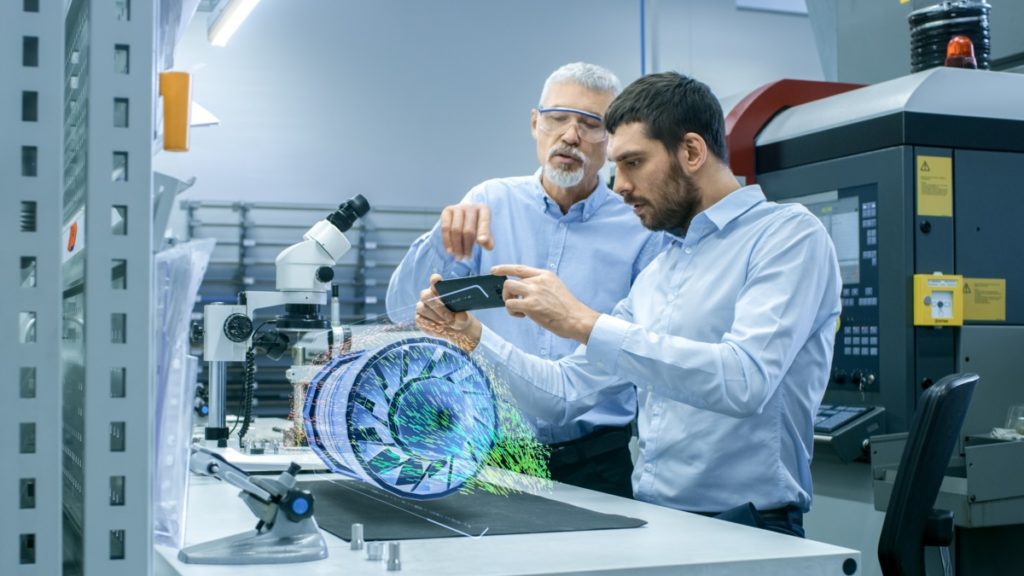
If your business hasn’t already embraced AI, you’re probably hearing a lot about it. You may have some questions. How does it work, and what are the rewards it can deliver? And perhaps most importantly: What’s involved in getting it up and running?
So, let’s look at what the process of implementing AI is like, and how to avoid the pitfalls to make sure it brings you a valuable return on investment.
What Is AI — and What Can It Do for You?
One simple way of looking at AI, or artificial intelligence, is that it’s a way of getting computers to do things that until recently we needed humans to do — at least where the subset of AI called machine learning is concerned.
Those tasks may involve things like combing through data to identify patterns (and deviations from expected patterns), predicting future events and trends, figuring out how to make good recommendations, or even learning how to read and write text and understand what’s happening in a photo.
Another way to look at it is that AI is the peak of a progression of data analysis techniques that get more complex and automated as you go up the scale. In a sense, all the advanced AI or analytics tools you hear about are just natural progressions of the more basic data and analytics concepts that you’re probably already familiar with.
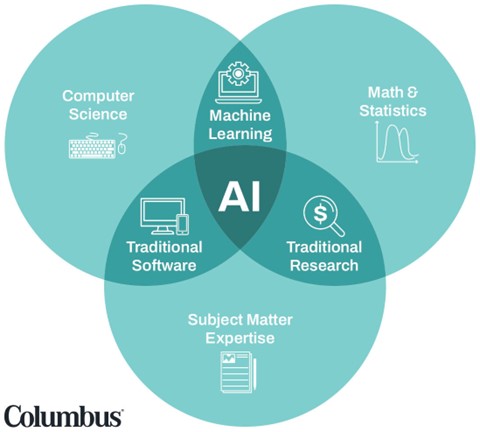
This Venn diagram shows you how AI sits at the intersection of traditional computer science techniques, traditional research and machine learning.
Here are just some of the benefits that AI can deliver when it’s successfully deployed:
- Better insights into business, customers and markets
- More precise estimates of costs
- Better demand forecasts
- A clearer picture of customers
- Identifying which customers are most likely to leave for a competitor
- The ability to optimize supply chains and other business processes
- Predictive maintenance — the ability to identify potential problems before they occur with equipment, and take preventive action
- Spotting fraud in transactions
For more on this, see How Artificial Intelligence is Used – and How It’s Reshaping Business.
Case Study: Improving Forecast Accuracy with AI
Let’s look at an example of an implementation that Columbus recently handled, for a manufacturing client that wanted to move its legacy ERP system to Microsoft Dynamics 365. One aspect of this worth noting is that the client’s existing setup included individual demand forecasts for more than 40,000 different factory items.
Fortunately, by using Azure Machine Learning and integrating with Dynamics 365, we were able to automate the selection of the best machine learning forecast model and then calculate and return those forecasts back to Dynamics 365 for additional demand planning.
By automating the model, we accomplished a couple of things. First, we allowed the data to guide us to the best model for each individual item, rather than having to hard code it the way they’d done in the old system. That automation is what made it possible to scale that forecasting across tens of thousands of individual factory items.
But additionally, allowing the data to guide the model creation and selection itself often led to significant improvements in forecast accuracy.
We were able to make a 9% improvement in the overall forecast error rate. That was a typical result for many of the individual factory items.
Getting Off the Ground: From Planning to Deployment
Any successful implementation of AI needs to begin by defining the goals and key business needs that will be addressed. And that leads to assessing what tools and data are required.
We generally start with an AI workshop. To home in on what kind of AI is needed, we start by looking at goals. Are we looking to grow the business or increase profitability? Is there a digital transformation under way? Based on questions like these, we can decide whether what we need is better information, or maybe better execution of business processes. And that in turn can bring us to the right set of AI tools for solving those needs.
After the AI workshop, we follow that up with a feasibility study to assess the existing infrastructure and the available data. This is essential to make sure that we have the data, resources, time and infrastructure to achieve our goals. Measure twice, cut once.
It can’t be emphasized enough that a successful AI implementation depends not just on having data, but having the right data. One of the most common pitfalls that can sink an AI project is data that’s incomplete, inconsistent, inaccessible, in the wrong format or just plain incorrect.
In some cases, it might be necessary to clean up data with pre-processing — reformatting, filling in missing values, correcting errors, etc. This is important because bad data leads to bad analysis, incorrect decisions and poor outcomes. It may be necessary to build some time for data wrangling into the process, to make sure everything’s ready to go.
After that, we can move to the stage where the magic happens: where we’re actually developing and training the models. This is an iterative process, as we assess results and make improvements, until we reach the point where the model is performing well enough that it’s ready for deployment.
People Are the Most Important Variable
From there, it’s important to have a solid plan not just for deploying the solution, but for integrating it into the everyday business processes of the company.
Unfortunately, this is where many AI projects end up failing to realize their value. Because the most challenging part of any AI implementation isn’t the technology itself: It’s getting the human piece of the puzzle in place.
Businesses sometimes overlook how difficult it can be for experienced employees to adapt to new ways of working — while other team members may be skeptical of new technology and the value it can deliver, or just confused about how to integrate new tools and processes into their daily tasks.
The bottom line is, if team members don’t receive the proper training and don’t see the benefits of changing their processes, they may simply stick to the way they’ve always done things, and your company won’t see the benefits of its investment in AI.
That means that in addition to investing in AI itself, businesses also need to invest in ensuring user adoption. For a look at some best practices in this area, see my article: A Barrier to AI Adoption: Getting Users Onboard to Maximize ROI.
And finally, there needs to be a plan in place for ongoing support. AI implementation isn’t a one-and-done execution that you set in motion and forget about. Data changes over time, as do customers, markets, environmental conditions and other values that you’ve based your model around.
You’ll need to pay attention to how the model is performing and retune periodically. Having a plan in place for what that will look like over six months, a year or three years will help make sure you’re building something that will keep delivering rewards into the future.
From Out-of-the-Box to Custom Solutions
What we’ve discussed above is a general framework that can be adapted to the needs of specific businesses. Some projects may have very limited goals or specific constraints, so we can always develop custom AI solutions. At the same time, there are many existing tools that that can be activated and deployed right off the shelf.
With that in mind, there are generally three levels of AI or machine learning complexity that we use to categorize these projects:
- built-in AI solutions
- managed AI solutions
- custom-built AI solutions
We may execute around AI tools that are already natively part of a platform, such as the AI or predictive analytics capabilities in a tool like Microsoft’s Power BI. There are plug-and-play components like Azure cognitive services, or natural language processing and computer vision that are part of Dynamics 365.
For other clients who need more tailored solutions, we may start from existing tools and capabilities and then develop more customized versions around that. In some cases, we may develop new tools that bring highly tailored capabilities that go beyond what’s contained in existing partner platforms or off-the-shelf systems.
Watch the Webinar for a Closer Look
Interested in learning more? Watch my recent webinar, where I explore this topic in greater detail: Webinar: Getting Started with AI